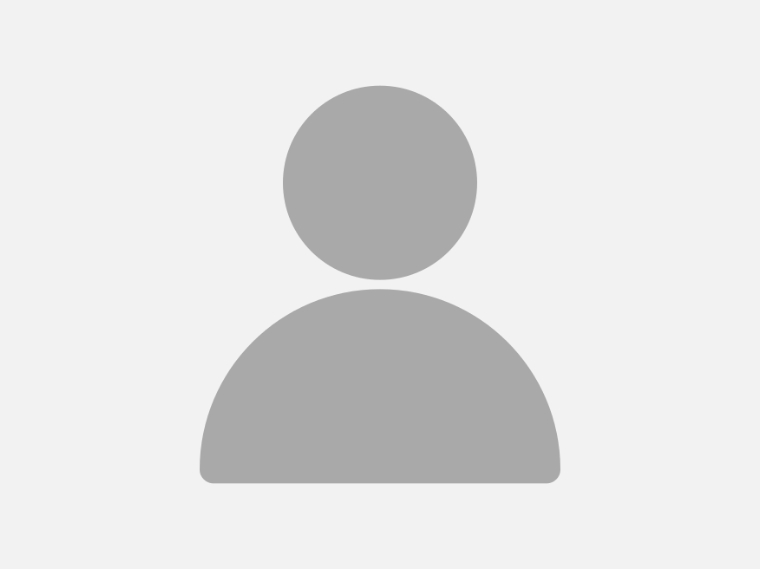
T
+34 946 567 842
F
+34 946 567 842
E
aperez@bcamath.org
Information of interest
- Orcid: 0000-0002-8128-1099
Postdoc Fellow at BCAM. The main methodological research lines include probabilistic graphical models, supervised classification, information theory, density estimation and feature subset selection. The methodological contributions have been applied to the fields of bioinformatics (genetics and epigenetics) and ecological modelling (fisheries).
-
Speeding-Up Evolutionary Algorithms to Solve Black-Box Optimization Problems
(2024-01-10)Population-based evolutionary algorithms are often considered when approaching computationally expensive black-box optimization problems. They employ a selection mechanism to choose the best solutions from a given population ...
-
Large-scale unsupervised spatio-temporal semantic analysis of vast regions from satellite images sequences
(2024)Temporal sequences of satellite images constitute a highly valuable and abundant resource for analyzing regions of interest. However, the automatic acquisition of knowledge on a large scale is a challenging task due to ...
-
Efficient Learning of Minimax Risk Classifiers in High Dimensions
(2023-08-01)High-dimensional data is common in multiple areas, such as health care and genomics, where the number of features can be tens of thousands. In such scenarios, the large number of features often leads to inefficient ...
-
Fast K-Medoids With the l_1-Norm
(2023-07-26)K-medoids clustering is one of the most popular techniques in exploratory data analysis. The most commonly used algorithms to deal with this problem are quadratic on the number of instances, n, and usually the quality of ...
-
Fast Computation of Cluster Validity Measures for Bregman Divergences and Benefits
(2023)Partitional clustering is one of the most relevant unsupervised learning and pattern recognition techniques. Unfortunately, one of the main drawbacks of these methodologies refer to the fact that the number of clusters is ...
-
Learning the progression patterns of treatments using a probabilistic generative model
(2022-12-15)Modeling a disease or the treatment of a patient has drawn much attention in recent years due to the vast amount of information that Electronic Health Records contain. This paper presents a probabilistic generative model ...
-
Implementing the Cumulative Difference Plot in the IOHanalyzer
(2022-07)The IOHanalyzer is a web-based framework that enables an easy visualization and comparison of the quality of stochastic optimization algorithms. IOHanalyzer offers several graphical and statistical tools analyze the results ...
-
An active adaptation strategy for streaming time series classification based on elastic similarity measures
(2022-05-21)In streaming time series classification problems, the goal is to predict the label associated to the most recently received observations over the stream according to a set of categorized reference patterns. In on-line ...
-
Generalized Maximum Entropy for Supervised Classification
(2022-04)The maximum entropy principle advocates to evaluate events’ probabilities using a distribution that maximizes entropy among those that satisfy certain expectations’ constraints. Such principle can be generalized for ...
-
Rank aggregation for non-stationary data streams
(2022)The problem of learning over non-stationary ranking streams arises naturally, particularly in recommender systems. The rankings represent the preferences of a population, and the non-stationarity means that the distribution ...
-
On the relative value of weak information of supervision for learning generative models: An empirical study
(2022)Weakly supervised learning is aimed to learn predictive models from partially supervised data, an easy-to-collect alternative to the costly standard full supervision. During the last decade, the research community has ...
-
LASSO for streaming data with adaptative filtering
(2022)Streaming data is ubiquitous in modern machine learning, and so the development of scalable algorithms to analyze this sort of information is a topic of current interest. On the other hand, the problem of l1-penalized ...
-
Are the statistical tests the best way to deal with the biomarker selection problem?
(2022)Statistical tests are a powerful set of tools when applied correctly, but unfortunately the extended misuse of them has caused great concern. Among many other applications, they are used in the detection of biomarkers so ...
-
On the use of the descriptive variable for enhancing the aggregation of crowdsourced labels
(2022)The use of crowdsourcing for annotating data has become a popular and cheap alternative to expert labelling. As a consequence, an aggregation task is required to combine the different labels provided and agree on a single ...
-
Machine learning from crowds using candidate set-based labelling
(2022)Crowdsourcing is a popular cheap alternative in machine learning for gathering information from a set of annotators. Learning from crowd-labelled data involves dealing with its inherent uncertainty and inconsistencies. In ...
-
Dirichlet process mixture models for non-stationary data streams
(2022)In recent years, we have seen a handful of work on inference algorithms over non-stationary data streams. Given their flexibility, Bayesian non-parametric models are a good candidate for these scenarios. However, reliable ...
-
Non-parametric discretization for probabilistic labeled data
(2022)Probabilistic label learning is a challenging task that arises from recent real-world problems within the weakly supervised classification framework. In this task algorithms have to deal with datasets where each instance ...
-
Comparing Two Samples Through Stochastic Dominance: A Graphical Approach
(2022)Nondeterministic measurements are common in real-world scenarios: the performance of a stochastic optimization algorithm or the total reward of a reinforcement learning agent in a chaotic environment are just two examples ...
-
Statistical assessment of experimental results: a graphical approach for comparing algorithms
(2021-08-25)Non-deterministic measurements are common in real-world scenarios: the performance of a stochastic optimization algorithm or the total reward of a reinforcement learning agent in a chaotic environment are just two examples ...
-
A cheap feature selection approach for the K -means algorithm
(2021-05)The increase in the number of features that need to be analyzed in a wide variety of areas, such as genome sequencing, computer vision or sensor networks, represents a challenge for the K-means algorithm. In this regard, ...
-
K-means for Evolving Data Streams
(2021-01-01)Nowadays, streaming data analysis has become a relevant area of research in machine learning. Most of the data streams available are unlabeled, and thus it is necessary to develop specific clustering techniques that take ...
-
On the fair comparison of optimization algorithms in different machines
(2021)An experimental comparison of two or more optimization algorithms requires the same computational resources to be assigned to each algorithm. When a maximum runtime is set as the stopping criterion, all algorithms need to ...
-
A Machine Learning Approach to Predict Healthcare Cost of Breast Cancer Patients
(2021)This paper presents a novel machine learning approach to per- form an early prediction of the healthcare cost of breast cancer patients. The learning phase of our prediction method considers the following two steps: i) in ...
-
Identifying common treatments from Electronic Health Records with missing information. An application to breast cancer.
(2020-12-29)The aim of this paper is to analyze the sequence of actions in the health system associated with a particular disease. In order to do that, using Electronic Health Records, we define a general methodology that allows us ...
-
Minimax Classification with 0-1 Loss and Performance Guarantees
(2020-12-01)Supervised classification techniques use training samples to find classification rules with small expected 0-1 loss. Conventional methods achieve efficient learning and out-of-sample generalization by minimizing surrogate ...
-
Statistical model for reproducibility in ranking-based feature selection
(2020-11-05)The stability of feature subset selection algorithms has become crucial in real-world problems due to the need for consistent experimental results across different replicates. Specifically, in this paper, we analyze the ...
-
General supervision via probabilistic transformations
(2020-08-01)Different types of training data have led to numerous schemes for supervised classification. Current learning techniques are tailored to one specific scheme and cannot handle general ensembles of training samples. This ...
-
Kernels of Mallows Models under the Hamming Distance for solving the Quadratic Assignment Problem
(2020-07)The Quadratic Assignment Problem (QAP) is a well-known permutation-based combinatorial optimization problem with real applications in industrial and logistics environments. Motivated by the challenge that this NP-hard ...
-
An efficient K-means clustering algorithm for tall data
(2020)The analysis of continously larger datasets is a task of major importance in a wide variety of scientific fields. Therefore, the development of efficient and parallel algorithms to perform such an analysis is a a crucial ...
-
An adaptive neuroevolution-based hyperheuristic
(2020)According to the No-Free-Lunch theorem, an algorithm that performs efficiently on any type of problem does not exist. In this sense, algorithms that exploit problem-specific knowledge usually outperform more generic ...
-
Supervised non-parametric discretization based on Kernel density estimation
(2019-12-19)Nowadays, machine learning algorithms can be found in many applications where the classifiers play a key role. In this context, discretizing continuous attributes is a common step previous to classification tasks, the main ...
-
Approaching the Quadratic Assignment Problem with Kernels of Mallows Models under the Hamming Distance
(2019-07)The Quadratic Assignment Problem (QAP) is a specially challenging permutation-based np-hard combinatorial optimization problem, since instances of size $n>40$ are seldom solved using exact methods. In this sense, many ...
-
On-line Elastic Similarity Measures for time series
(2019-04)The way similarity is measured among time series is of paramount importance in many data mining and machine learning tasks. For instance, Elastic Similarity Measures are widely used to determine whether two time series are ...
-
On the evaluation and selection of classifier learning algorithms with crowdsourced data
(2019-02-16)In many current problems, the actual class of the instances, the ground truth, is unavail- able. Instead, with the intention of learning a model, the labels can be crowdsourced by harvesting them from different annotators. ...
-
Predictive engineering and optimization of tryptophan metabolism in yeast through a combination of mechanistic and machine learning models
(2019)In combination with advanced mechanistic modeling and the generation of high-quality multi-dimensional data sets, machine learning is becoming an integral part of understanding and engineering living systems. Here we show ...
-
Crowd Learning with Candidate Labeling: an EM-based Solution
(2018-09-27)Crowdsourcing is widely used nowadays in machine learning for data labeling. Although in the traditional case annotators are asked to provide a single label for each instance, novel approaches allow annotators, in case ...
-
Are the artificially generated instances uniform in terms of difficulty?
(2018-06)In the field of evolutionary computation, it is usual to generate artificial benchmarks of instances that are used as a test-bed to determine the performance of the algorithms at hand. In this context, a recent work on ...
-
On-Line Dynamic Time Warping for Streaming Time Series
(2017-09)Dynamic Time Warping is a well-known measure of dissimilarity between time series. Due to its flexibility to deal with non-linear distortions along the time axis, this measure has been widely utilized in machine learning ...
-
Nature-inspired approaches for distance metric learning in multivariate time series classification
(2017-07)The applicability of time series data mining in many different fields has motivated the scientific community to focus on the development of new methods towards improving the performance of the classifiers over this particular ...
-
An efficient approximation to the K-means clustering for Massive Data
(2017-02-01)Due to the progressive growth of the amount of data available in a wide variety of scientific fields, it has become more difficult to manipulate and analyze such information. In spite of its dependency on the initial ...
-
Nature-inspired approaches for distance metric learning in multivariate time series classification
(2017)The applicability of time series data mining in many different fields has motivated the scientific community to focus on the development of new methods towards improving the performance of the classifiers over this particular ...
-
Efficient approximation of probability distributions with k-order decomposable models
(2016-07)During the last decades several learning algorithms have been proposed to learn probability distributions based on decomposable models. Some of these algorithms can be used to search for a maximum likelihood decomposable ...
-
An efficient approximation to the K-means clustering for Massive Data
(2016-06-28)Due to the progressive growth of the amount of data available in a wide variety of scientific fields, it has become more difficult to manipulate and analyze such information. In spite of its dependency on the initial ...
-
Efficient approximation of probability distributions with k-order decomposable models
(2016-01-01)During the last decades several learning algorithms have been proposed to learn probability distributions based on decomposable models. Some of these algorithms can be used to search for a maximum likelihood decomposable ...
MixtureDecModels
Learning mixture of decomposable models with hidden variables
Authors: Aritz Pérez
License: free and open source software
Placement
Local
BayesianTree
Approximating probability distributions with mixtures of decomposable models
Authors: Aritz Pérez
License: free and open source software
Placement
Local
KmeansLandscape
Study the k-means problem from a local optimization perspective
Authors: Aritz Pérez
License: free and open source software
Placement
Local
PGM
Procedures for learning probabilistic graphical models
Authors: Aritz Pérez
License: free and open source software
Placement
Local
On-line Elastic Similarity Measures
Adaptation of the most frequantly used elastic similarity measures: Dynamic Time Warping (DTW), Edit Distance (Edit), Edit Distance for Real Sequences (EDR) and Edit Distance with Real Penalty (ERP) to on-line setting.
Authors: Izaskun Oregi, Aritz Perez, Javier Del Ser, Jose A. Lozano
License: free and open source software
MRCpy: a library for Minimax Risk Classifiers
MRCpy library implements minimax risk classifiers (MRCs) that are based on robust risk minimization and can utilize 0-1-loss.
Authors: Kartheek Reddy, Claudia Guerrero, Aritz Perez, Santiago Mazuelas
License: free and open source software
OPTECOT - Optimal Evaluation Cost Tracking
This repository contains supplementary material for the paper Speeding-up Evolutionary Algorithms to solve Black-Box Optimization Problems. In this work, we have presented OPTECOT (Optimal Evaluation Cost Tracking): a technique to reduce the cost of solving a computationally expensive black-box optimization problem using population-based algorithms, avoiding loss of solution quality. OPTECOT requires a set of approximate objective functions of different costs and accuracies, obtained by modifying a strategic parameter in the definition of the original function. The proposal allows the selection of the lowest cost approximation with the trade-off between cost and accuracy in real time during the algorithm execution. To solve an optimization problem different from those addressed in the paper, the repository also contains a library to apply OPTECOT with the CMA-ES (Covariance Matrix Adaptation Evolution Strategy) optimization algorithm.
Authors: Judith Echevarrieta, Etor Arza, Aritz Pérez
License: free and open source software
TransfHH
A multi-domain methodology to analyze an optimization problem set
Authors: Etor Arza, Ekhiñe Irurozki, Josu Ceberio, Aritz Perez
License: free and open source software
FractalTree
Implementation of the procedures presented in A. Pérez, I. Inza and J.A. Lozano (2016). Efficient approximation of probability distributions with k-order decomposable models. International Journal of Approximate Reasoning 74, 58-87.
Authors: Aritz Pérez
License: free and open source software