Development of novel mathematical and experimental methodologies to control neuronal activity and dissect spatio-temporal neuronal codes
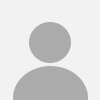
Objective:
Subproject 2 is divided in three different lines: phase response functions, estimation of model parameters and synaptic conductances and mapping multi-time scale models to macroscopic descriptions. These lines focus on crucial aspects of the overall coordinated project and are completely aligned with the aim of introducing novel mathematical techniques to unveil, with the help of numerical computations and experiments, invariant laws of brain processes and architecture seen as a multi-scale dynamical system. Under the term of phase response functions we deploy challenging problems related to the sensitivity of neurons and networks of neurons to external stimuli, as well as synchronisation problems. Phase response functions are a powerful tool to study synchrony of limit cycle oscillators in that they predicting phase advancement induced by specific stimuli. We have pioneered the mathematical extension of this concept to transient states and we now plan to exploit them in realistic situations. In this topic, we are interested in: 1. Connecting the concept of phase sensitivity of oscillating networks of neurons with the excitability properties encoded in the intrinsic channels of the neurons; 2. Studying synchronisation of population of neurons under this approach in order to understand how different rhythmic activities in the brain interact, that is, what is the information that the phase response functions carry in network models and how can it explain mechanisms underlying brain phenomena; 3. Apply control theory techniques in order to force the dynamics of neuron oscillators to a follow a prescribed behaviour (e.g., drug administration protocols). The second line faces two important neuroscience challenges involving inference of unknown parameters: model identification and connectivity estimation. On one side, experimental data cannot be associated to a specific mathematical neuron model without a careful electrophysiological protocol, which is often unfeasible. Therefore, heuristic methods to either estimate free parameters of generic biophysical models of neurons or to obtain black boxes that identify the dynamics of recorded data are extremely suitable to make this neuron-to-model match. We will tackle this problem following two approaches: Bayesian inference methods and wavelet artificial neural networks. On the other side, we will also deal with the question of disambiguating cells that present similar dynamics. Concerning connectivity estimation, we will address the problem of estimating the local connectivity of a neuron even in highly nonlinear regimes and, most importantly, start a thorough experimental closed-loop protocols (together with members of Subproject 1 and other collaborators) to validate the estimation methods we have developed so far. Finally, the third line will focus on mapping multi-time scale models to macroscopic descriptions, that is, analyzing models coarse-grained models of the activity of populations of neurons. Our aim is understanding how oscillations emerge (in strong connection with the 1st line) and detecting complex oscillations induced either by the presence of different time scales or by the topology of the network itself.