New paper from BCAM researcher in the Journal of Machine Learning Research
Ikerbasque Asssociate researcher Santiago Mazuelas has published the paper “Minimax Risk Cassifiers with 0-1 Loss” on the prestigious Journal of Machine Learning Research (JMLR)
Santiago Mazuelas, Ikerbasque Research Associate in the Basque Center for Applied Mathematics - BCAM's Data Science area has recently published the paper Minimax Risk Classifiers with 0-1 Loss in the Journal of Machine Learning Research (JMLR). JMLR is arguably the most prestigious journal in the field of Machine Learning with an acceptance rate below 20% and an editorial board composed by the most distinguished researchers in the field, including Alan Turing awardees and the Vice President of Google Research, among others.
The paper Minimax Risk Classifiers with 0-1 Loss has been developed under an international collaboration with Mauricio Romero from the Federal University of Bahia (Brazil) and Peter Grünwald from the National Research Institute for Mathematics and Computer Science (Netherlands). Prof. Romero is an Associate Professor specialized in convex optimization that was a Visiting Fellow in BCAM in 2021. Prof. Grünwald is a Full Professor in Leiden University, head of the machine learning group in CWI, recipient of the Van Dantzig Prize in 2010, and the President of the Association for Computational Learning. The international research collaboration that has resulted in the published paper has been led by Santiago Mazuelas who is the first author of the paper.
The paper presents Minimax Risk Classifiers (MRCs) that minimize the worst-case 0-1 loss over general classification rules and provide tight performance guarantees. It shows how the out-of-sample performance of MRCs can be reliably estimated at learning, and that the MRCs are strongly universally consistent using rich feature mappings. Differently from existing methods, the proposed methodology can offer classification techniques that do not rely on specific training samples and choices for surrogate losses/hypothesis classes. Instead, MRC learning is based on expectation estimates, and its inductive bias comes only from a feature mapping that determines which expectations are estimated. Therefore, the methods presented can provide techniques that are robust to practical situations that defy common assumptions, e.g., training samples that follow a different distribution or display heavy tails. The machine learning group at BCAM has already utilized such methodology for multiple important applications including the prognosis of COVID-19 infections, and the prediction of energy consumption and prize.
The published paper has been developed under a research effort undertaken over several years by Santiago Mazuelas together with his collaborators and students. This research endeavor is developing a novel methodology for supervised classification that goes beyond the conventional empirical risk minimization (ERM) approach and obtains classification rules that minimize worst-case error probabilities. The results obtained by the group in the last three years have resulted in over six publications in the most prestigious journals and conferences in the field, including NeurIPs, ICML, and JMLR. These papers have been authorized by three senior researchers at BCAM (Santiago Mazuelas, Aritz Perez, and Jose A. Lozano), three PhD students at BCAM (Veronica Alvarez, Jose Segovia, and Kartheek Reddy), and four international collaborators (Peter Grünwald from the Netherlands, Yuan Shen from China, Anqi Liu from the United States, and Mauricio Romero from Brazil). In the coming years the group will continue pursuing this research line expanding the team of students and international collaborators, while maintaining the publications quality.
Santiago Mazuelas received the Ph.D. in Mathematics and Ph.D. in Telecommunications Engineering from the University of Valladolid, Spain, in 2009 and 2011, respectively. He is currently an Ikerbasque Research Associate at the Basque Center for Applied Mathematics (BCAM). Prior to joining BCAM, he was a Staff Engineer at Qualcomm Corporate Research and Development from 2014 to 2017. He previously worked from 2009 to 2014 as Postdoctoral Fellow and Associate in the Laboratory for Information and Decision Systems (LIDS) at the Massachusetts Institute of Technology (MIT). His general research interest is the application of mathematics to solve practical problems, currently his work is primarily focused on machine learning and statistical signal processing. He has received the Young Scientist Prize from the Union Radio-Scientifique Internationale (URSI) Symposium in 2007, and the Early Achievement Award from the IEEE ComSoc in 2018. His papers received the IEEE Communications Society Fred W. Ellersick Prize in 2012, the SEIO-FBBVA Best Applied Contribution in the Statistics Field in 2022, and Best Paper Awards from the IEEE ICC in 2013, the IEEE ICUWB in 2011, and the IEEE Globecom in 2011.
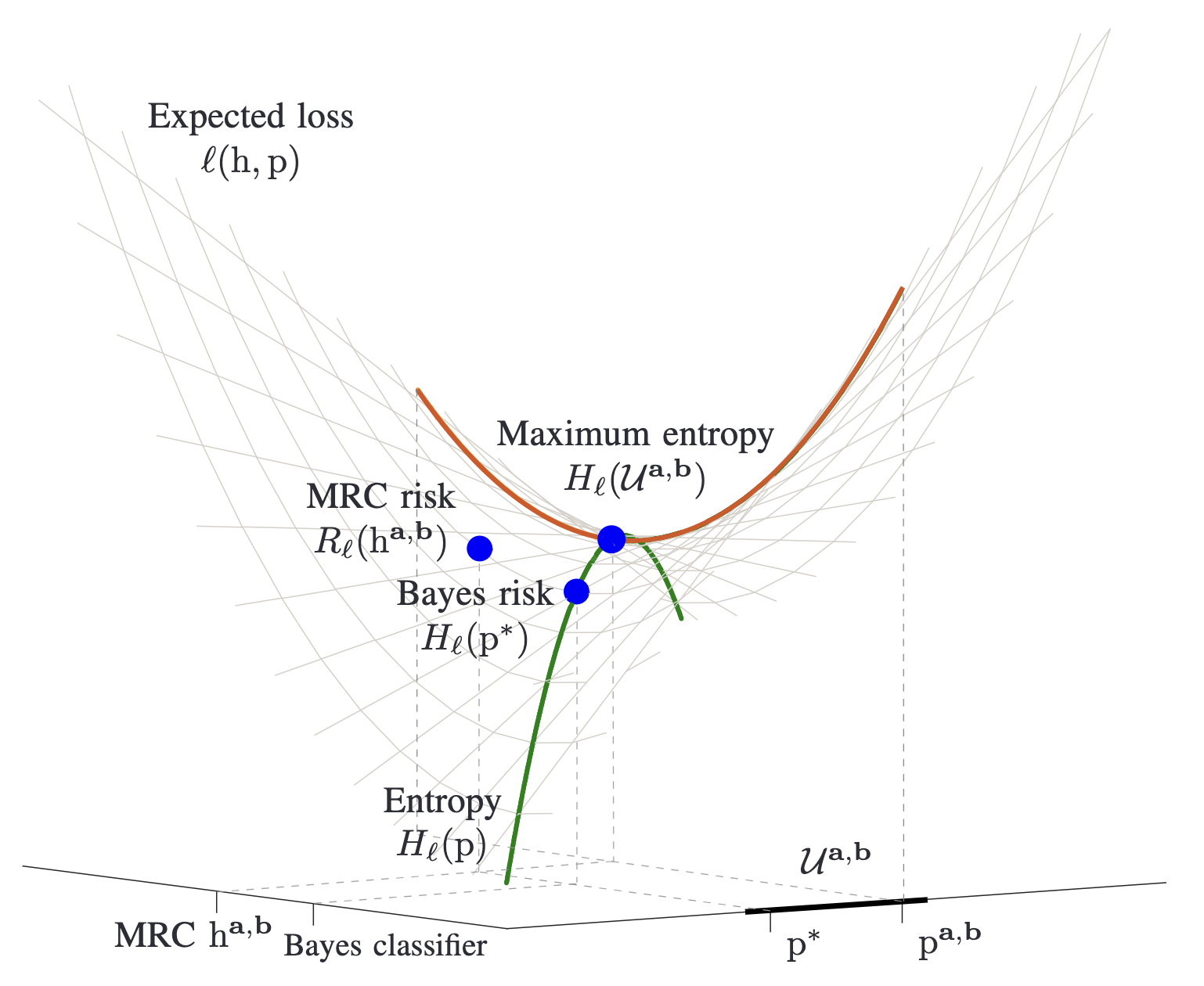
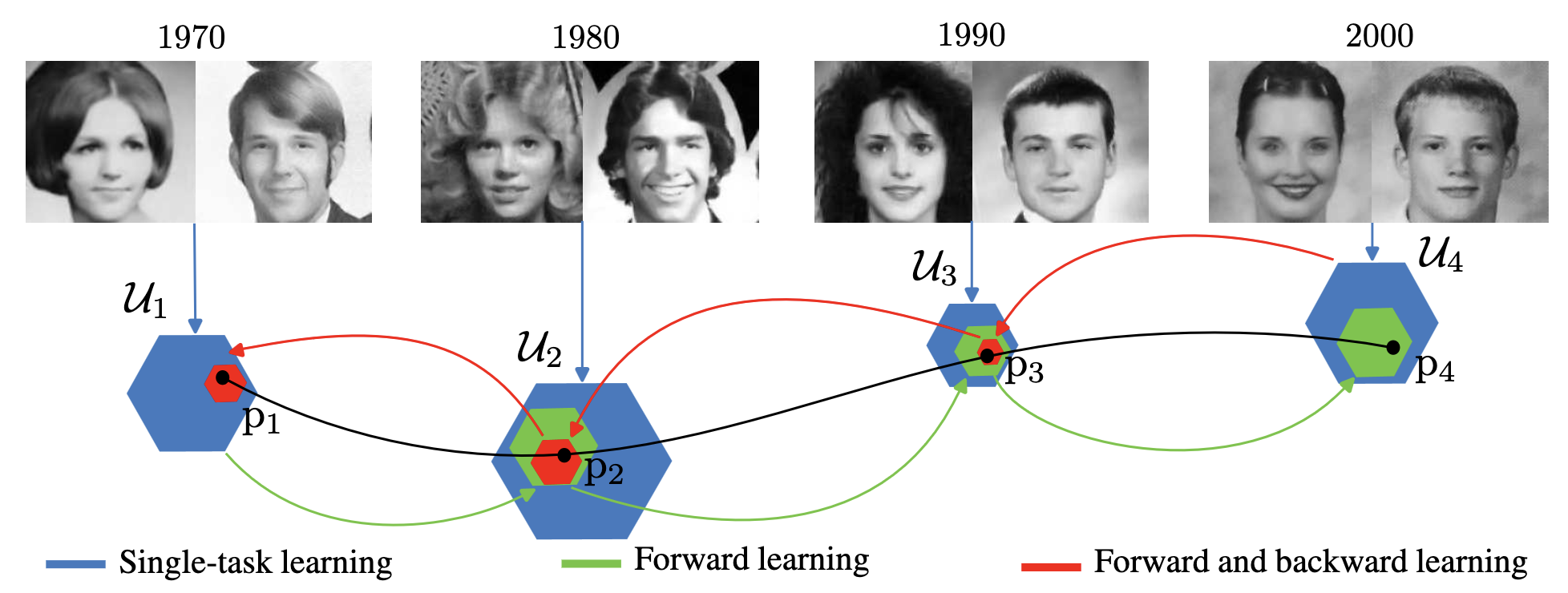
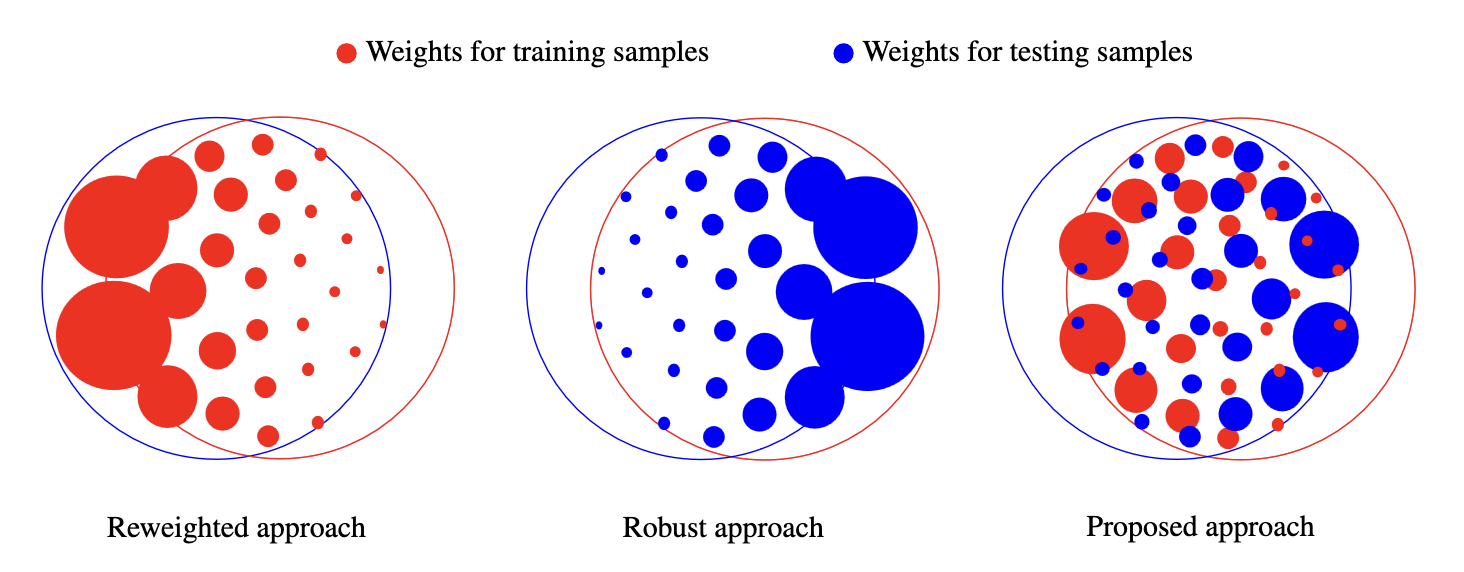
Related news
About the center