AUTOEV@L, the future of mobility
The project is a strategic commitment by the R&D centres and entities of the ACBC, universities and intermediate technological agents to strengthen collaborations and generate synergies
AUTOEV@L is a Collaborative Fundamental Research Project, within the ELKARTEK 2021 call, for the technological evolution towards multi-vehicle automation and evaluation of highly automated driving functions. The project is part of the strategy already presented in the AUTOLIB project of the ELKAERTEK 2019 call. It is being developed by an interdisciplinary consortium of eight entities including CEIT, Ikerlan, Tecnalia, Vicomtech, AIC - Automotive Intelligence Center, Mondragon Unibertsitatea, University of the Basque Country - UPV/HU and Basque Center for Applied Mathematics - BCAM.
The Machine Learning group at BCAM, will focus its research in the development of machine learning algorithms for accurate localisation of autonomous vehicles that use collaborative information efficiently and obtain high accuracies in complex environments with obstacles.
Autonomous driving requires techniques for precise vehicle localisation. Global navigation satellite systems (GNSS) can meet the demand for outdoor navigation, but are unreliable indoors and in urban environments due to the strong attenuation of satellite signals. Therefore, numerous location solutions based on other technologies, such as radio frequency, have been developed in recent years.
However, in practice, the range of RF techniques is affected by non-line-of-sight (NLOS) propagation. The NLOS condition is very common in autonomous driving, as urban environments often consist of numerous elements that make the driving scenario a complex learning problem. In particular, NLOS conditions can lead to large range errors that significantly degrade localisation performance.
Specifically, each vehicle obtains estimates of its position using measurements with respect to GNSS satellites and other vehicles, identifying the cases where NLOS signal transmission occurs and mitigating the potential errors that such data generates.
The proposed NLOS mitigation method will be based on self-training or weak monitoring, thus eliminating the need for labelled data and the associated cost of obtaining it. Furthermore, the self-training procedure does not need to train additional trainees. Such a method is integrated into a multisensory information fusion localisation system, as illustrated in Figure 1. The figure on the right shows the advantage gained by integrating information relating to distance measurements (represented by circular arcs in blue) with angular information (represented by green straight lines). The left figure shows how this approximation of position can be further improved by incorporating contextual information, such as knowing that we are in a particular car park.
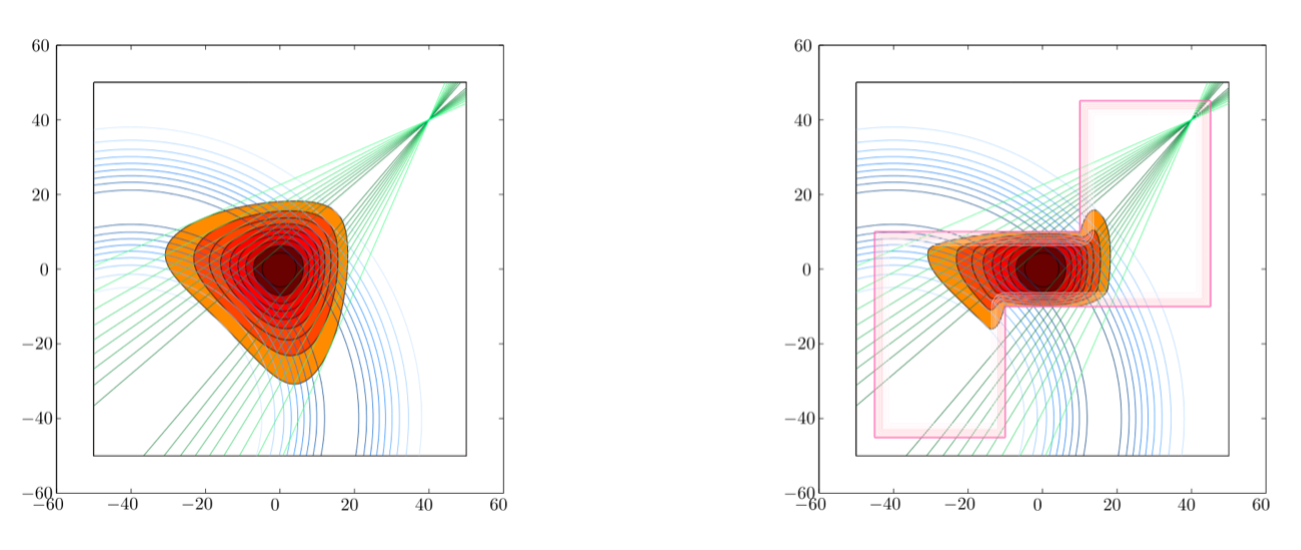
Figure 1. Information fusion. The left figure shows the fusion of weak location information and weak angular information. The right figure shows the fusion of weak location information, weak angular information and weak contextual information.
Expected results
Methods under development include multi-sensor information fusion and NLOS mitigation to improve accuracy. In addition, they do not require labelled data and do not rely on fingerprinting, avoiding the need to build expensive databases. In this way, the methods under development will improve the process of vehicle localisation, with a special emphasis on improved localisation, navigation in complex environments with obstacles and a further step in improving autonomous driving.
Related news
About the center