MATHDES
Mathematical Design, Modelling and Simulations
Mathematical Design, Modeling, and Simulations (MATHDES) group works on the design, analysis, implementation, and optimization of numerical schemes for mathematical models arising from real-life applications.
Principal Investigators:
Michael Barton and David Pardo
Our research spans areas of Deep Learning, Inverse Problems, Finite Elements, Massive Computations, Numerical Analysis, Geometric Modeling, Computer Aided Design, and Modeling of Manufacturing Processes. We work in close interaction with industrial partners and institutions to promote transfer of knowledge and obtain feedback from real-life applications.
We currently work on three European Projects:
(a) a FET-OPEN project on Analysis, Design, and Manufacturing using Microstructures ADAM^2, which aims at revolutionizing the design-analysis-manufacturing pipeline, coordinated by Michael Barton; (b) a Marie Curie RISE Action MATHROCKS, intended to improve and exchange interdisciplinary knowledge on applied mathematics, high performance computing, and geophysics, coordinated by David Pardo; and (c) the European NextGenerationEU project IA4TES, funded by the Spanish Ministry for Economic Affairs and Digital Transition and led by Iberdrola, for structural health monitoring and assessment of remaining useful life of offshore wind platforms.
We collaborate with multiple prestigious institutions and industrial partners, including The University of Texas at Austin (USA), Stratasys LTD (Israel), INRIA (France), Hutchinson S.A. (France), Seoul National University (South Korea), Technion - Israel Institute of Technology (Israel), MacQuaire University (Australia), Ecole Polytechnique Federale de Lausanne (Switzerland), Curtin University (Australia), Technical University of Wein (Austria), King Abdullah University of Science and Technology (Saudi Arabia), Software Competence Center Hagenberg (Austria), Pontifical Catholic University of Valparaíso (Chile), Pontifical Catholic University of Chile, National University of Colombia, Central University of Venezuela, University of Buenos Aires (Argentina), Barcelona Supercomputing Center (Spain), Polytechnic University of Catalunya (Spain), University of Barcelona (Spain), University Charles III of Madrid (Spain), Tecnalia (Spain), Trimek S.A. (Spain), and University of the Basque Country (UPV/EHU).
This BCAM group has strong collaboration ties with the sister UPV/EHU Group MATHMODE.
In particular, we are currently involved in the following research projects:
Deep Learning Algorithms for Inverse Problems:
We study and develop Deep Learning methods to interpret (invert) in real-time elasto-acoustic and electromagnetic measurements. To achieve this objective, we design and implement proper Deep Neural Network architectures, loss functions, and error control algorithms enabling us to efficiently approximate physically meaningful inverse solutions.
Massive Finite Element Simulations:
We develop a set of numerical Galerkin-based methods for producing massive synthetic datasets for training Deep Neural Networks. Some examples include variants of Isogeometric Analysis, Dimensionality Reduction Algorithms, Reduced Order Models, Proper Generalized Decompositions, and hp-Adaptivity.
Geometric Modeling:
We model manufacturing processes such as 5-axis Computer Numerically Controlled (CNC) machining, see Fig.1, hot-wire cutting, or hybrid (additive and subtractive) manufacturing.

Fig. 1: 5-axis flank machinability of a reference surface (dark) using various machining tools (green). The tool-paths that meet fine machining tolerances are shown as ruled surface (motions of the tool axis, yellow).
Manufacturing Applications:
In collaboration with the High Performance Manufacturing Group (UPV/EHU), we design path-planning algorithms for multi-axis CNC machining. An example of a simulation of 5-axis flank milling with a custom-shaped tool is shown in Fig. 2. The tool and its motion are both unknowns in our optimization-based framework. Manufactured result (blisk) machined with our algorithm using a conical tool is shown in Fig. 3.
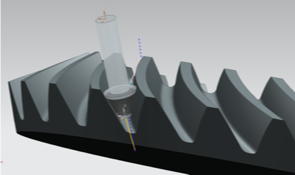
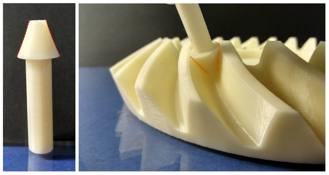
Fig. 2: Simulation of path-planning for 5-axis double-flank CNC machining of a spiral bevel gear using a custom-shaped milling tool, (left). The 3D-printed prototype of the designed tool is shown in right.
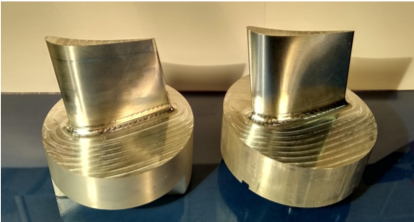
Fig. 3: Finishing of the blisk blade using the state-of-the-art software (Siemens NX, left) and our algorithm (right). Additionally, to higher accuracy (not visible), observe the smooth light reflection in our result, that is due to negligible distance error between the neighboring milling paths.
Geophysical applications:
We employ advanced numerical simulation and inversion methods to characterize the Earth´s subsurface, see Fig. 4. This is critical in several applications, such as (a) the prospecting of water, precious minerals, and hydrocarbons, (b) earthquake prediction and seismic hazard estimation, (c) seismic monitoring, (d) mine detection, (e) geothermal energy production, and (f) management of CO2 sequestration at the industrial scale that is needed to abate the global climate change problem.
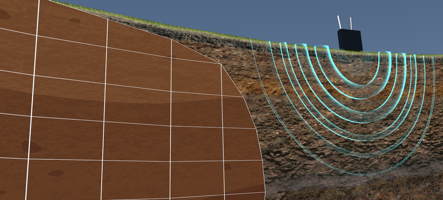
Fig. 4: Controlled Source Electromagnetic Measurements for Earth Exploration
Offshore Wind Energy application:
We design Deep Neural Network architectures as a support of the design and operation of emerging technologies for offshore wind energy applications in three areas: (a) for accelerating CFD simulations using OpenFoam for analysing the hydrodynamics of support structures; (b) for structural health monitoring of components (mooring systems, power cables) in offshore floating platforms (see Fig. 5).; (c) real-time control of offshore wind energy farms for Fault Detection and Fault Tolerant Control.
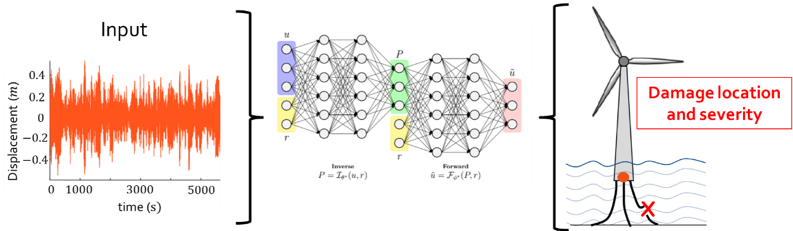
Fig. 5: Autoencoder architecture for Structural Health Monitoring of Floating offshore wind components
High-quality smooth finishing of blade-like geometries via $G^1$ multi-pass 5-axis flank CNC machining using conical cutting tools
Rajain, K.; Gomez Escudero, G.; Bizzarri, M.; Gonzalez-Barrio, H.; Calleja, A.; López de Lacalle, L.N.; Barton, M. (2024-12-04)
Existing multi-pass planning methods often result in undesirable gaps or overlaps between adjacent paths of a cutter. These gaps and/or overlaps in the path-planning stage cause artifacts in the physical machining and these ...
Mixed-precision finite element kernels and assembly: Rounding error analysis and hardware acceleration
Croci, M.; Wells, G. N. (2024-10-16)
In this paper we develop the first fine-grained rounding error analysis of finite element (FE) cell kernels and assembly. The theory includes mixed-precision implementations and accounts for hardware-acceleration via matrix ...
Reducing spatial discretization error on coarse CFD simulations using an openFOAM-embedded deep learning framework
Gonzalez-Sieiro, J.; Pardo, D.; Nava, V.; Calo, V.M.; Towara, M. (2024-09-09)
We propose a method for reducing the spatial discretization error of coarse computational fluid dynamics (CFD) problems by enhancing the quality of low-resolution simulations using deep learning. We feed the model with fine-...
On tiling spherical triangles into quadratic subpatches
Vrsek, J.; Bizzarri, M.; Lavicka, M.; Barton, M.; Kosinka, J. (2024-06-01)
Various interpolation and approximation methods arising in several practical applications in geometric modeling deal, at a particular step, with the problem of computing suitable rational patches (of low degree) on the unit ...
Damage identification in bridges combining deep learning and computational mechanic
CL file
Datasets (Zenodo) for the paper "Manufacturing of screw rotors via 5-axis double-flank CNC machining"
Authors: Michael Barton, Michal Bizzarri
License: Zenodo
CL file
Datasets (Zenodo) for the paper "Geometry and tool motion planning for curvature adapted CNC machining"
Authors: Michael Barton, Michal Bizzarri, Oleksii Sliusarenko, Florian Rist, Helmut Pottmann
License: Zenodo