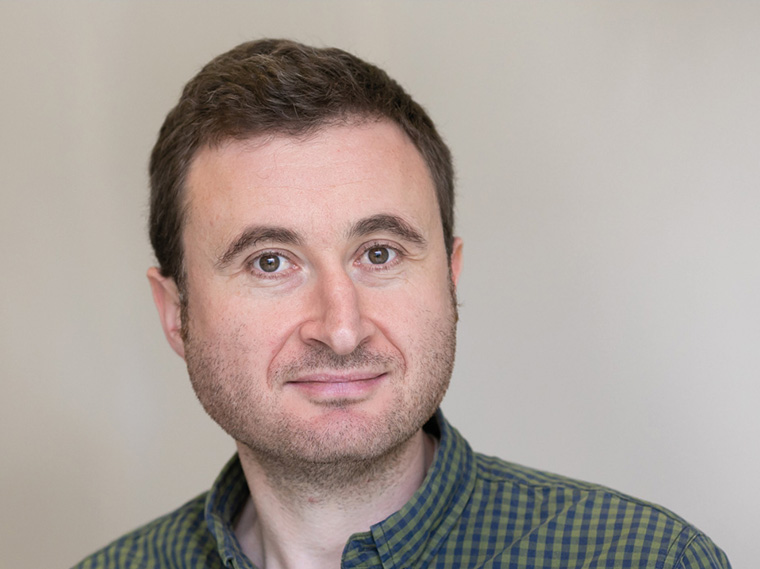
T
+34 946 567 842
F
+34 946 567 842
E
smazuelas@bcamath.org
Information of interest
Santiago Mazuelas received the Ph.D. in Mathematics and Ph.D. in Telecommunications Engineering from the University of Valladolid, Spain, in 2009 and 2011, respectively.
He is currently an Ikerbasque Research Associate at the Basque Center for Applied Mathematics (BCAM). Prior to joining BCAM, he was a Staff Engineer at Qualcomm Corporate Research and Development from 2014 to 2017. He previously worked from 2009 to 2014 as Postdoctoral Fellow and Associate in the Laboratory for Information and Decision Systems (LIDS) at the Massachusetts Institute of Technology (MIT). His general research interest is the application of mathematics to solve practical problems, currently his work is primarily focused on machine learning methods.
Dr. Mazuelas is Editor for the IEEE Transactions on Wireless Communications and was Area Editor (signal processing) for the IEEE Communications Letters from 2017 to 2022. He has served as Technical Program Vice-chair at the 2021 IEEE Globecom, and as Symposium Co-chair at several IEEE ICC and Globecom conferences. He received the Young Scientist Prize from the Union Radio-Scientifique Internationale (URSI) Symposium in 2007, and the Early Achievement Award from the IEEE ComSoc in 2018. His papers received the IEEE Communications Society Fred W. Ellersick Prize in 2012, the SEIO-FBBVA Best Applied Contribution in the Statistics Field in
2022, and Best Paper Awards from the IEEE ICC in 2013, the IEEE ICUWB in 2011, and the IEEE Globecom in 2011.
-
A Unified View of Double-Weighting for Marginal Distribution Shift
(2025-03)Supervised classification traditionally assumes that training and testing samples are drawn from the same underlying distribution. However, practical scenarios are often affected by distribution shifts, such as covariate ...
-
Supervised Learning with Evolving Tasks and Performance Guarantees
(2025-01-09)Multiple supervised learning scenarios are composed by a sequence of classification tasks. For instance, multi-task learning and continual learning aim to learn a sequence of tasks that is either fixed or grows over time. ...
-
TDOA-based localization under uniform prior knowledge: Performance bounds and its efficient calculation
(2025-01-01)The emergence of a myriad of location-based services has imposed a key role on wireless localization systems. The accuracy of such systems can be enhanced by using prior information on the target location area, commonly ...
-
Minimax Risk Classifiers for Mislabeled Data: a Study on Patient Outcome Prediction Tasks
(2025)Healthcare datasets are often impacted by incorrect or mislabeled data, due to imperfect an- notations, data collection problems, ambiguity, and subjective interpretations. Incorrectly classified data, referred to as ...
-
Multi-task Online Learning for Probabilistic Load Forecasting
(2024-11)Load forecasting is essential for the efficient, reliable, and cost-effective management of power systems. Load forecasting performance can be improved by learning the similarities among multiple entities (e.g., regions, ...
-
Minimax Forward and Backward Learning of Evolving Tasks with Performance Guarantees
(2023-12)For a sequence of classification tasks that arrive over time, it is common that tasks are evolving in the sense that consecutive tasks often have a higher similarity. The incremental learning of a growing sequence of ...
-
Efficient Learning of Minimax Risk Classifiers in High Dimensions
(2023-08-01)High-dimensional data is common in multiple areas, such as health care and genomics, where the number of features can be tens of thousands. In such scenarios, the large number of features often leads to inefficient ...
-
Minimax Risk Classifiers with 0-1 Loss
(2023-07-01)Supervised classification techniques use training samples to learn a classification rule with small expected 0 -1 loss (error probability). Conventional methods enable tractable learning and provide out-of-sample ...
-
Indoor Localization System With NLOS Mitigation Based on Self-Training
(2023-07-01)Location-awareness has become a fundamental requirement for multiple emerging applications with the rapid development of wireless technologies. The high-accuracy ranging enabled by ultra-wide bandwidth (UWB) signals is ...
-
Double-Weighting for Covariate Shift Adaptation
(2023-07)Supervised learning is often affected by a covariate shift in which the marginal distributions of instances (covariates $x$) of training and testing samples $p_\text{tr}(x)$ and $p_\text{te}(x)$ are different but the label ...
-
A Variational Learning Approach for Concurrent Distance Estimation and Environmental Identification
(2023-02-01)Wireless propagated signals encapsulate rich information for high-accuracy localization and environment sensing. However, the full exploitation of positional and environmental features as well as their correlation remains ...
-
Female Models in AI and the Fight Against COVID-19
(2022-11-01)Gender imbalance has persisted over time and is well documented in science, technology, engineering and mathematics (STEM) and singularly in artificial intelligence (AI). In this article we emphasize the importance of ...
-
The role of asymmetric prediction losses in smart charging of electric vehicles
(2022-07-01)Climate change prompts humanity to look for decarbonisation opportunities, and a viable option is to supply electric vehicles with renewable energy. The stochastic nature of charging demand and renewable generation requires ...
-
Minimax Classification under Concept Drift with Multidimensional Adaptation and Performance Guarantees
(2022-07)The statistical characteristics of instance-label pairs often change with time in practical scenarios of supervised classification. Conventional learning techniques adapt to such concept drift accounting for a scalar rate ...
-
Variational Bayesian Framework for Advanced Image Generation with Domain-Related Variables
(2022-05-23)Deep generative models (DGMs) and their conditional counterparts provide a powerful ability for general-purpose generative modeling of data distributions. However, it remains challenging for existing methods to address ...
-
Generalized Maximum Entropy for Supervised Classification
(2022-04)The maximum entropy principle advocates to evaluate events’ probabilities using a distribution that maximizes entropy among those that satisfy certain expectations’ constraints. Such principle can be generalized for ...
-
A Deep Learning Approach for Generating Soft Range Information from RF Data
(2022-01-24)Radio frequency (RF)-based techniques are widely adopted for indoor localization despite the challenges in extracting sufficient information from measurements. Soft range information (SRI) offers a promising alternative ...
-
Derivation of a Cost-Sensitive COVID-19 Mortality Risk Indicator Using a Multistart Framework
(2022-01-14)The overall global death rate for COVID-19 patients has escalated to 2.13% after more than a year of worldwide spread. Despite strong research on the infection pathogenesis, the molecular mechanisms involved in a fatal ...
-
A Semi-Supervised Learning Approach for Ranging Error Mitigation Based on UWB Waveform
(2021-12-30)Localization systems based on ultra-wide band (UWB) measurements can have unsatisfactory performance in harsh environments due to the presence of non-line-of-sight (NLOS) errors. Learning-based methods for error ...
-
Deep GEM-based network for weakly supervised UWB ranging error mitigation
(2021-12-30)Ultra-wideband (UWB)-based techniques, while becoming mainstream approaches for high-accurate positioning, tend to be challenged by ranging bias in harsh environments. The emerging learning-based methods for error ...
-
Deep Generative Model for Simultaneous Range Error Mitigation and Environment Identification
(2021-12-07)Received waveforms contain rich information for both range information and environment semantics. However, its full potential is hard to exploit under multipath and non-line- of-sight conditions. This paper proposes a ...
-
Location Awareness in Beyond 5G Networks
(2021-11-01)Location awareness is essential for enabling contextual services and for improving network management in 5th generation (5G) and beyond 5G (B5G) networks. This paper provides an overview of the expanding opportunities ...
-
Minimax Classification with 0-1 Loss and Performance Guarantees
(2020-12-01)Supervised classification techniques use training samples to find classification rules with small expected 0-1 loss. Conventional methods achieve efficient learning and out-of-sample generalization by minimizing surrogate ...
-
General supervision via probabilistic transformations
(2020-08-01)Different types of training data have led to numerous schemes for supervised classification. Current learning techniques are tailored to one specific scheme and cannot handle general ensembles of training samples. This ...
-
Probabilistic Load Forecasting Based on Adaptive Online Learning
(2020)Load forecasting is crucial for multiple energy management tasks such as scheduling generation capacity, planning supply and demand, and minimizing energy trade costs. Such relevance has increased even more in recent ...
-
Soft information for localization-of-things
(2019-11-01)Location awareness is vital for emerging Internetof- Things applications and opens a new era for Localizationof- Things. This paper first reviews the classical localization techniques based on single-value metrics, such ...
-
Crowd-Centric Counting via Unsupervised Learning
(2019-07-11)Counting targets (people or things) within a moni-tored area is an important task in emerging wireless applications,including those for smart environments, safety, and security.Conventional device-free radio-based ...
-
Belief Condensation Filtering For Rssi-Based State Estimation In Indoor Localization
(2019)Recent advancements in signal processing and communication systems have resulted in evolution of an intriguing concept referred to as Internet of Things (IoT). By embracing the IoT evolution, there has been a surge of ...
-
Spatiotemporal information coupling in network navigation
(2018-12)Network navigation, encompassing both spatial and temporal cooperation to locate mobile agents, is a key enabler for numerous emerging location-based applications. In such cooperative networks, the positional information ...
-
Soft range information for network localization
(2018-06-15)The demand for accurate localization in complex environments continues to increase despite the difficulty in extracting positional information from measurements. Conventional range-based localization approaches rely on ...
MRCpy: a library for Minimax Risk Classifiers
MRCpy library implements minimax risk classifiers (MRCs) that are based on robust risk minimization and can utilize 0-1-loss.
Authors: Kartheek Reddy, Claudia Guerrero, Aritz Perez, Santiago Mazuelas
License: free and open source software